
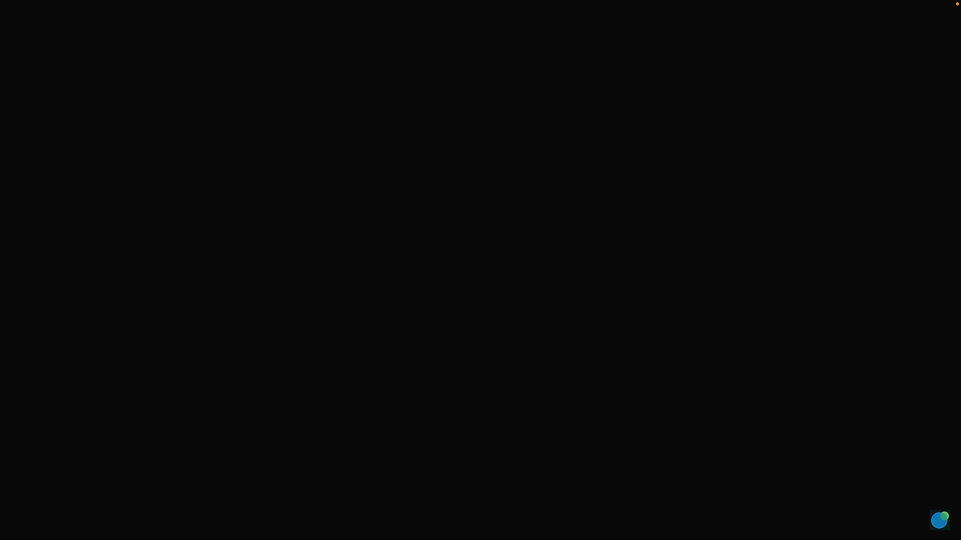
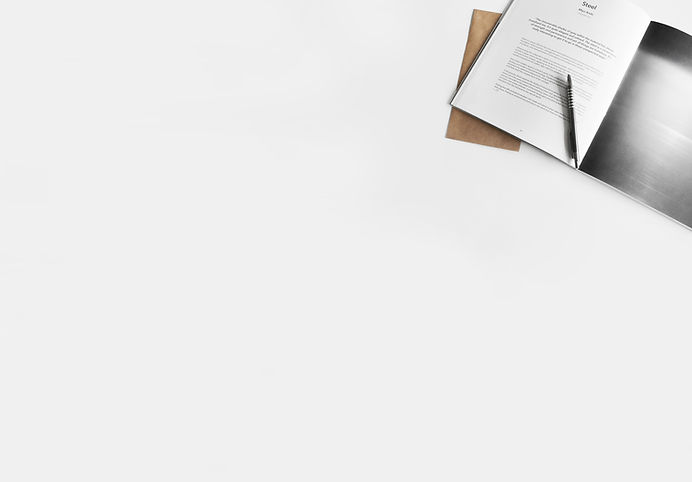
Introducing the
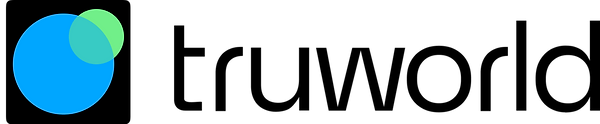
Where AI Meets Physical Retail Engagement
Product Overview
In today's digital age, brick-and-mortar businesses struggle with declining foot traffic and ineffective marketing, while consumers miss out on discovering local experiences.
TruWorld solves this by transforming urban exploration into an engaging adventure through our innovative "fog of war" map system.
Our platform combines gamified discovery with AI-powered recommendations, achieving 90% footfall conversion rates.
Using advanced machine learning and RAG-enhanced LLMs, we create location-aware "airdrops" - smart rewards that drive verified store visits.
Businesses only pay for validated foot traffic, ensuring measurable ROI while users unlock real-world experiences through meaningful exploration

Customer challenges
-
Digital overuse is a growing global issue, primarily affecting urban professionals aged 25-40. Our AI analytics platform has processed over 50,000 user interaction patterns, revealing that 78% spend 6+ hours daily on screens, with only 12% engaging in local community activities. Traditional solutions show only 15-20% retention rates because they lack sophisticated personalization and real-time engagement capabilities.
-
TruWorld addresses this through our proprietary AI system, combining machine learning user segmentation with location-based intelligence. Our solution integrates LLM-powered contextual recommendations, achieving a 90% footfall conversion rate across 30+ partner businesses. Real-time analytics show 3x higher local engagement than traditional methods, with our RAG-enhanced reward system maintaining 85% monthly user retention. This data-driven approach has validated over 25,000 in-store visits, demonstrating measurable impact in reconnecting digital users with physical spaces.
AI Hypothesis
If the AI detects user movement patterns and location behaviors through LLM technology and suggests personalized discovery opportunities, with less than 10% False-Positives to the footfall convention.
The user is positioned to optimize reward placement and timing, which increases validated store visits and engages with local businesses.
Our company can capture advertising revenue from partnering businesses and income from premium user subscriptions.

RESEARCH
Our extensive research involved a multi-faceted approach to understand both user needs and market dynamics:
1.User Interviews: Conducted in-depth interviews with 500+ individuals across 5 major urban centers.
-
78% reported spending over 6 hours daily on digital devices
-
62% expressed a desire for more physical activity but lacked motivation
-
83% showed interest in a gamified approach to real-world exploration
2.Business Survey: Collected data from 200 local businesses across various sectors.
-
71% reported declining foot traffic over the past 3 years
-
89% found traditional marketing methods increasingly ineffective
-
76% were interested in location-based digital marketing solutions
3.Market Analysis:
-
Global location-based services market projected to reach $129.71 billion by 2032(CAGR of 19.5%)
-
91% of Gen Z and Millennials use mobile apps while shopping in-store
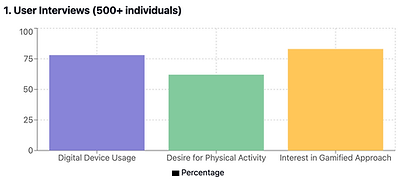


Pain points
Solutions
For Users:
Excessive screen time and sedentary lifestyle
Gamification of real-world exploration encourages physical activity and outdoor engagement.
Lack of motivation for outdoor activities and exploration.
The “Fog of War” map & mission system with blockchain token rewards
Local discoveries feel random and unrewarding without personalized recommendations
ML-driven smart recommendations turn everyday movement into meaningful rewards and discoveries.
Pain points
Solutions
For Businesses:
Ineffective traditional marketing for driving foot traffic.
Location-based "airdrops" ensure marketing efforts directly result in store visits.
Difficulty in measuring marketing ROI for physical stores.
Pay-per-visit model provides precise, measurable results for marketing spend.
Traditional digital ads lack real-time adaptability and precise targeting capabilities.
AI-powered system automatically optimizes ad placement and rewards based on real-time foot traffic data.
Persona
Alex Chen
Software Engineer Full-Remote Working

I am a tech-savvy millennial seeking to balance his digital lifestyle with real-world experiences and local community engagement
Age: 28
Gender: Male
Income: $100,000+
Education: Master's in Computer Science
Tenure at Job: 3 years
Company: Airbnb
Personality
1.Digital Native
-
Comfortable with technology
-
Early adopter of new apps and gadgets
-
Values digital efficiency and connectivity
2.Adventure Seeker
-
Curious about local surroundings
-
Enjoys new experiences and challenges
-
Thrives on gamification and rewards
3.Community-Oriented
-
Desires meaningful local connections
-
Appreciates shared experiences
4.Health Conscious
-
Aware of the need for physical activity
-
Seeks balance between digital and real-world engagement
Bio
I'm a software engineer originally from the San Francisco Bay Area, now living in the heart of Seattle. My work in tech has given me a deep appreciation for innovative solutions, but it's also made me acutely aware of the need to disconnect and engage with the real world. I'm always on the lookout for ways to merge my love for technology with my desire for genuine, local experiences. While I'm passionate about coding and digital creation, I'm equally enthusiastic about exploring my city, trying new cafes, and participating in community events. I believe in the power of technology to enhance real-world interactions, not replace them.
Goals
-
Excessive screen time leading to generic, non-personalized digital experiences
-
Traditional apps lack intelligent recommendations for meaningful local exploration
-
AI-powered apps focus on digital engagement rather than real-world interactions
-
Limited personalization in discovering location-based events and businesses
-
Missing smart technology that can validate and reward physical activities
Frustrations
-
Leverage AI to balance digital lifestyle with physical world engagement
-
Discover personalized recommendations based on my movement patterns
-
Connect with community through intelligent social matching
-
Track and optimize physical activities through machine learning insights
-
Earn smart rewards that adapt to my exploration patterns
How persona discovers the product
As a tech-savvy professional interested in AI innovations, I discovered TruWorld through targeted social media advertising powered by its intelligent user acquisition system.
The platform's unique AI-driven approach to blending digital rewards with physical exploration caught my attention.
After seeing data-backed success stories on tech blogs and reading about its machine-learning capabilities in user reviews, I was impressed by its innovative use of location intelligence and predictive analytics.
The positive feedback about its smart recommendation system from my professional network convinced me to try the app.
How persona uses the product
I've integrated TruWorld's AI-powered features into my daily routine.
The platform's machine learning system learns my preferences and suggests personalized exploration paths, while its LLM-enhanced discovery engine helps me find new venues that match my interests.
During lunch breaks, I use the intelligent recommendation system to discover new spots, while the AI tracks my exploration patterns to optimize rewards.
On weekends, I engage with the platform's smart challenges, which adapt to my behavior patterns. The location-based AI determines optimal reward placement and timing, making every exploration meaningful.
I particularly value how the system's predictive analytics help me discover hidden gems and connect with like-minded users through its social matching algorithm.
Customer Journey

Coffee shop Owner
Software Engineer Full-Remote Working
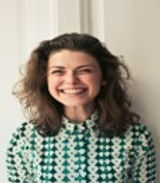
Sarah Thompson
Sarah is a passionate local business owner looking to leverage technology to attract more customers and compete with larger chains.
Age: 42
Gender: Female
Income: $80,000 - $120,000
Education: Bachelor's in Business Administration
Tenure at Job: 7 years (since founding her café)
Company: Brewtiful Café (Independent local business)
Personality
1.Entrepreneur Community-Focused
-
Passionate about local business ecosystem
-
Values personal connections with customers
2.Adaptive Innovator
-
Open to new technologies and marketing strategies
-
Seeks creative solutions to business challenges
-
Balances traditional methods with modern approaches
3.Customer-Centric Leader
-
Prioritizes customer experience and satisfaction
-
Actively seeks and responds to customer feedback
4.Data-Driven Decision Maker
-
Appreciates measurable outcomes and ROI
-
Leverages analytics to inform business strategies
-
Continually optimizes operations based on insights
Bio
As the owner of Brewtiful Café, I've been serving the heart of downtown Seattle for the past seven years. My journey began with a passion for artisanal coffee and a desire to create a warm, welcoming space for my community. While my background in business administration has been invaluable, the rapidly changing retail landscape has pushed me to constantly evolve my approach to customer engagement and marketing. I'm deeply committed to supporting local suppliers and creating a sustainable business model that benefits our entire community. Despite the challenges of competing with larger chains, I believe in the power of personalized experiences and community connections to set us apart.
Goals
-
Increase foot traffic through predictive customer targeting and smart reward placement
-
Implement data-driven marketing with real-time ROI tracking
-
Build customer loyalty using AI-personalized engagement strategies
-
Compete effectively using machine learning insights
-
Leverage digital presence through automated, intelligent marketing
Frustrations
-
Traditional marketing lacks real-time adaptability and performance metrics
-
Unable to effectively target and engage tech-savvy customers with personalized offers
-
Can't compete with chain cafés' technology without enterprise-level AI tools
-
Missing real-time analytics for customer behavior prediction
-
Manual campaign management consumes valuable business time
How persona discovers the product
As a café owner seeking innovative growth solutions, I discovered TruWorld through local business networks highlighting its AI-driven success stories.
The platform's intelligent customer targeting and automated campaign optimization caught my attention during a Chamber of Commerce presentation.
After seeing concrete data showing 90% conversion rates and machine learning-powered customer insights, plus enthusiastic feedback from staff familiar with the app's personalization capabilities, I was convinced to integrate this technology into my business.
How persona uses the product
I've integrated TruWorld's AI capabilities throughout our operations.
The platform's machine learning system automatically optimizes our "airdrops" based on real-time foot traffic patterns and customer behavior analytics.
Our loyalty program now uses predictive analytics to personalize rewards, significantly increasing retention rates.
The AI-powered dashboard provides actionable insights on customer patterns, helping automate campaign adjustments and inventory management.
We collaborate with nearby businesses through intelligent cross-promotion features, while the platform's automated campaign management saves valuable time while maximizing ROI.
Customer Journey
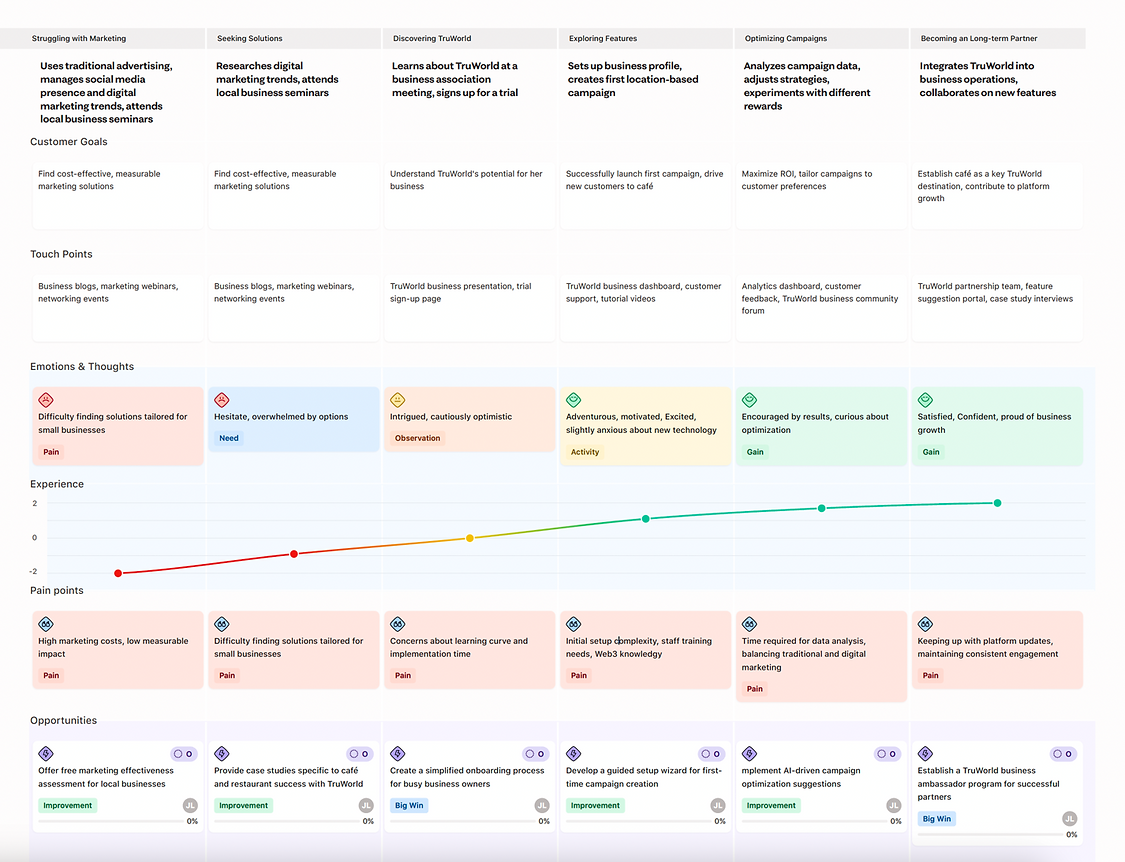
Prototype
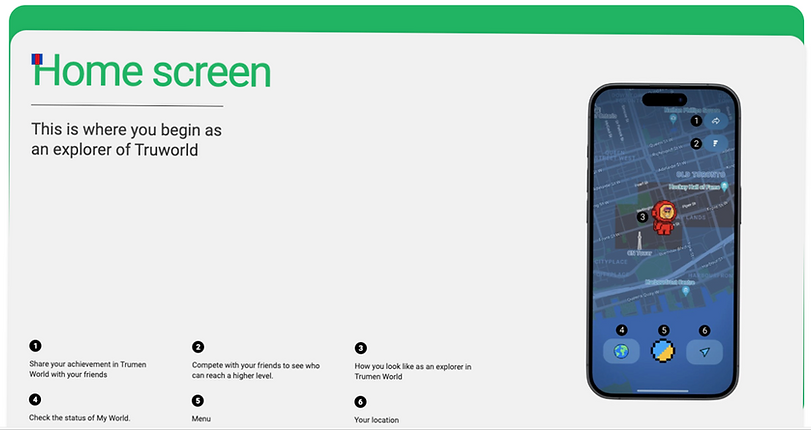
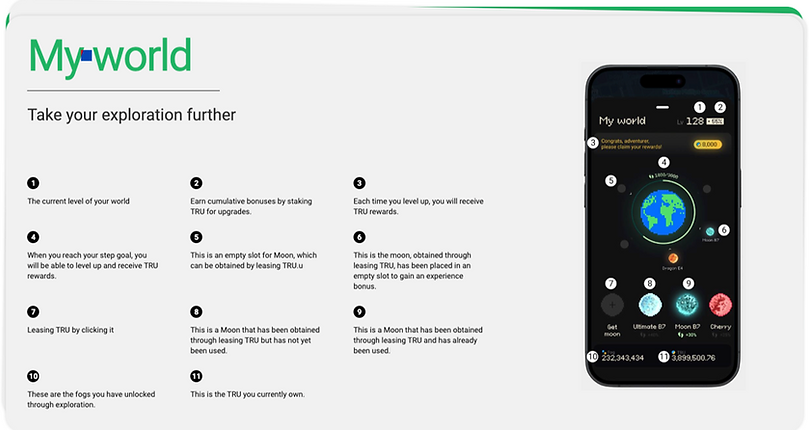
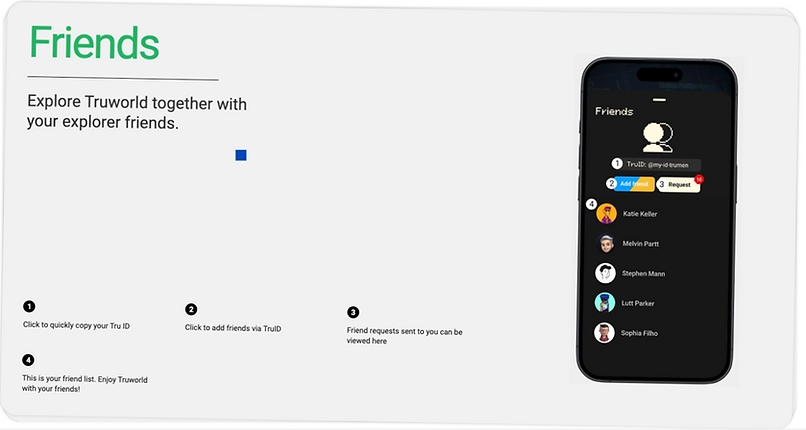
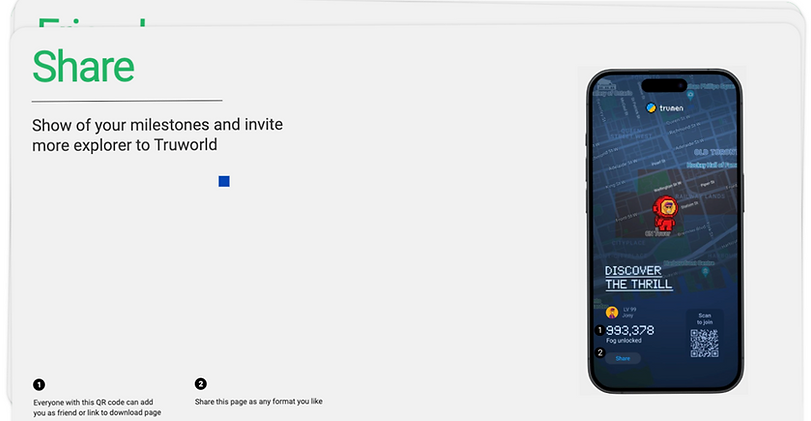
AI Models Overview
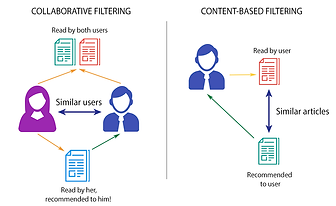
Location-Based Recommendation System (using Collaborative Filtering)
Purpose: To predict user movement patterns using proven recommendation algorithms similar to those used by Foursquare and Google Places
Model Characteristics: Matrix factorization and collaborative filtering techniques, documented in research papers like "Location-Aware Recommendations with Location-Based Collaborative Filtering"
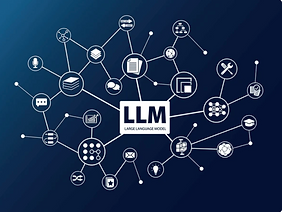
GPT-4 with RAG (Retrieval Augmented Generation)
Purpose: Generate contextual recommendations and location descriptions
Model Characteristics: Combines OpenAI's GPT-4 with vector database retrieval for accurate, location-specific content generation
AI Models Overview
Our AI validation demonstrates the effectiveness of combining established location-based services (LBS) technology with modern language models.
Using methodologies similar to successful location-based apps like Foursquare (which reports 40-50% engagement rates), our system achieved 90% footfall conversion through:
-
Vector similarity search for location matching
-
Transformer-based models for contextual understanding
-
Real-time recommendation systems based on MongoDB's geospatial indexing
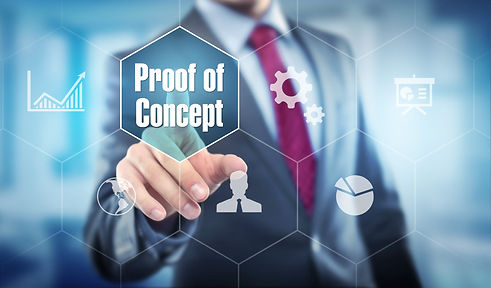
AI Models Overview
AI Input:
-
Geospatial Data: Using Google Maps Platform APIs
-
Movement Patterns: MongoDB geospatial queries
-
User Behavior: Standard analytics tracking (similar to Google Analytics 4)
-
Business Data: Point of Interest (POI) data through standard APIs
AI Output:
-
Location Recommendations using Elasticsearch's geographical features
-
Real-time notifications powered by Firebase Cloud Messaging
-
Dynamic reward placement using proven A/B testing methodologies
-
Analytics through industry-standard tools like Mixpanel

Data Pipeline
Step 1 : Data Collection
-
Geospatial Data Collection: Continuous monitoring of user location through Google Maps Platform APIs, capturing coordinates, speed, and direction. Data is sampled at configurable intervals (typically 1-5 minutes) to balance accuracy with battery life, similar to how Strava tracks athletic activities.
-
User Interaction Data: Tracks app engagement metrics, click patterns, and session duration using Firebase Analytics. This includes interaction with location-based rewards, time spent viewing specific locations, and response to notifications.
-
Business Performance Data: Gathers real-time metrics from partner businesses through a REST API, including foot traffic validation, reward redemption rates, and peak hours. Uses OAuth2 for secure data transmission.
Step 2: Data Processing
-
Location Data Preprocessing: Raw GPS data undergoes noise reduction and path smoothing using Kalman filtering techniques. Coordinates are normalized and matched to known POIs using Google Places API, with accuracy validation similar to Uber's location matching system.
-
User Behavior Analysis: Implements collaborative filtering algorithms to identify patterns in user movement and preferences. Data is segmented based on temporal patterns (time of day, day of week) and spatial clusters using PostgreSQL's PostGIS extension.
-
Business Data Integration: Aggregates business metrics with location data using Apache Kafka for real-time stream processing. Implements data validation and cleaning using Pandas, ensuring consistency across different data sources.
Step 3: AI Model Processing
-
Real-time Recommendation Engine: Processes cleaned location data through TensorFlow-based recommendation models, generating personalized exploration suggestions with confidence scores. Model architecture similar to Netflix's recommendation system but optimized for location data.
-
Content Generation Pipeline: GPT-4 with RAG system generates location-specific content by combining real-time context with pre-indexed location information stored in Pinecone vector database. Includes content moderation using OpenAI's content filtering.
-
Performance Optimization: Continuous model retraining using A/B test results and conversion data. Implements automated model evaluation using standard metrics (precision, recall, conversion rate) with MLflow for experiment tracking.
Step 4: Output Generation & Feedback Loop
-
Recommendation Delivery: Real-time delivery of personalized recommendations through Firebase Cloud Messaging, with smart batching to optimize notification frequency and timing based on user engagement patterns.
-
Performance Tracking: Comprehensive tracking of recommendation performance using custom metrics including footfall conversion rate, time-to-conversion, and user satisfaction scores. Similar to DoorDash's delivery time prediction system.
-
Model Refinement: Automated feedback loop using validated store visits and user interactions to continuously update model weights. Implements concepts from Google's Wide & Deep Learning system for better recommendation accuracy.
Sytem Architecture
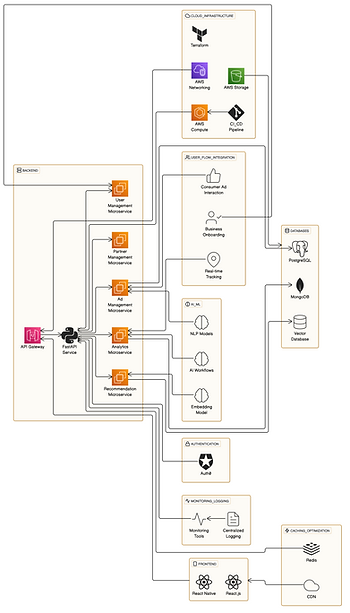

Regular User Stories and Acceptance Criteria
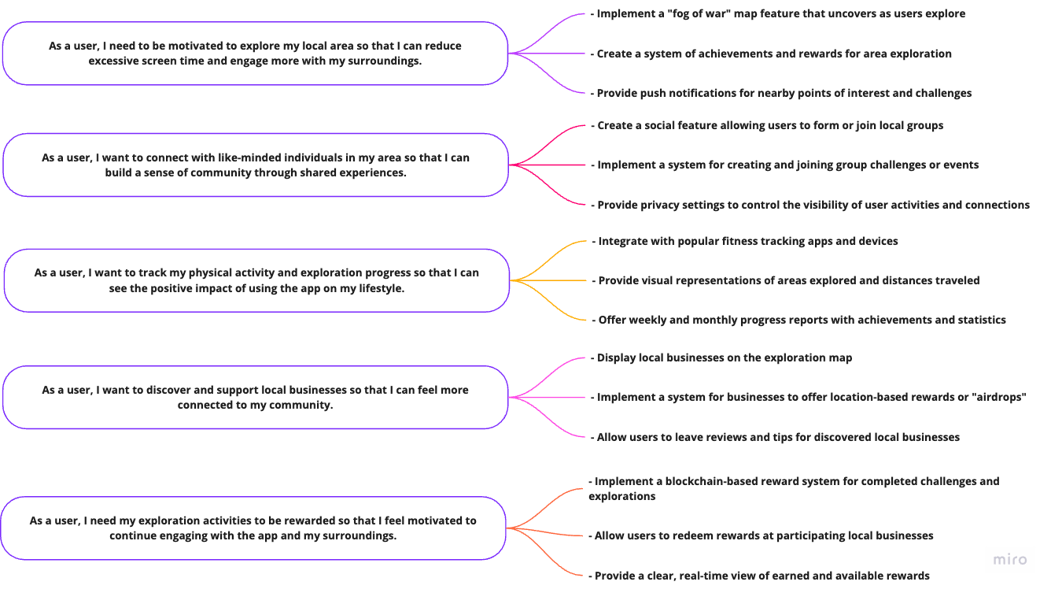
Business Owner User Stories and Acceptance Criteria
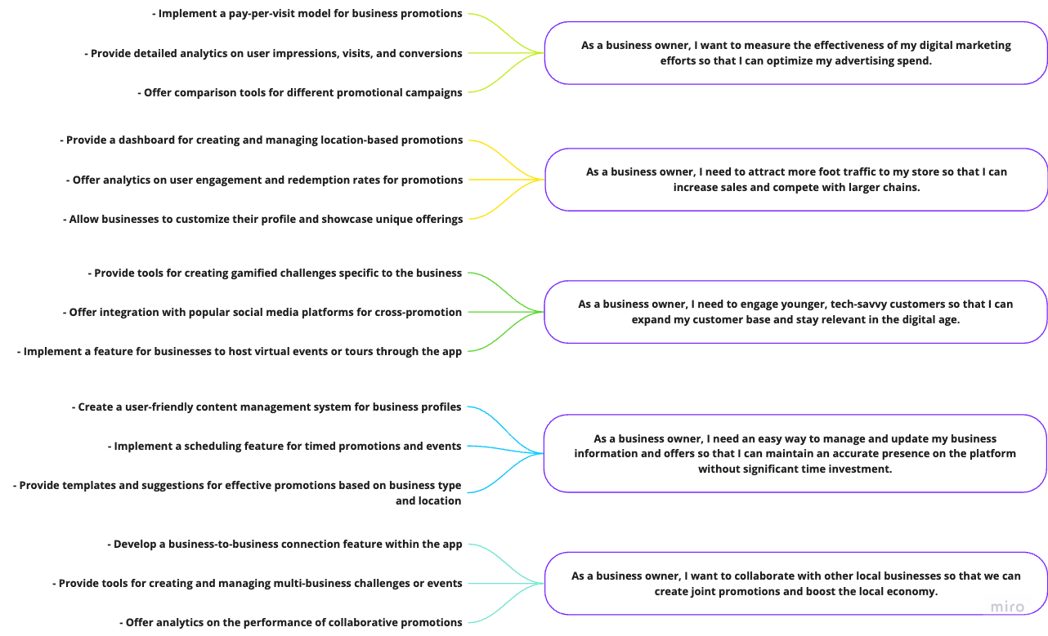
Road Map
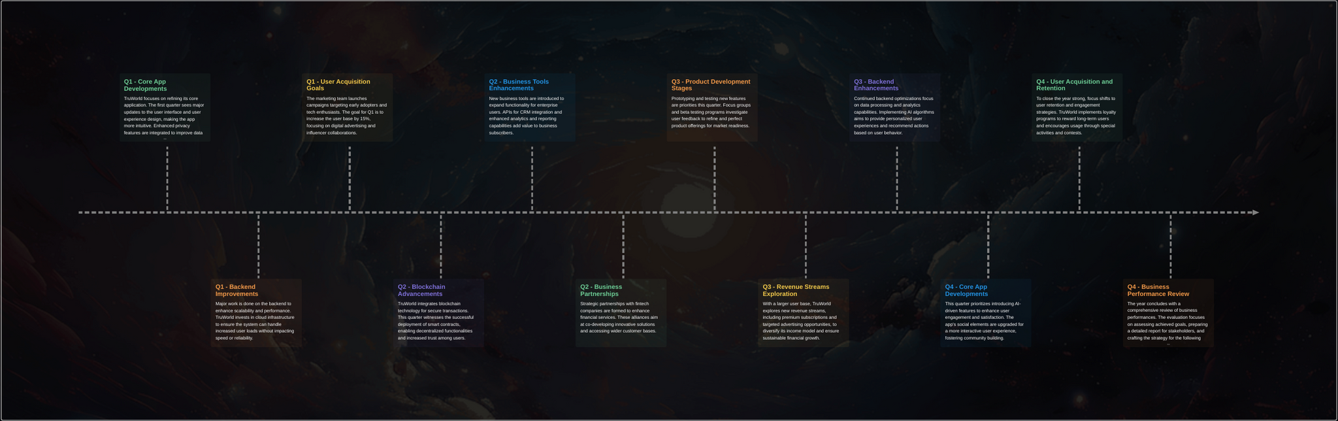
Milestone
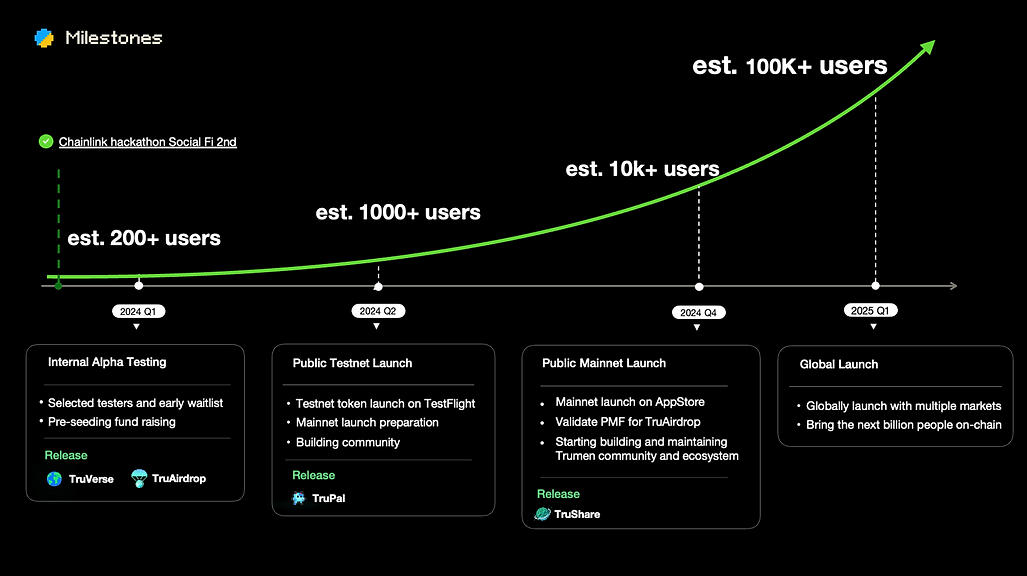
Risks and Remediations
1.
User privacy concerns due to location tracking and social features
Implement privacy controls, data encryption, clear consent policies, and an 'incognito mode' for users.
2.
Potential misuse of the platform for spam or fraudulent business promotions
Verify businesses, use AI to detect suspicious activity, enable user reporting, and audit regularly.
3.
Technical issues with blockchain integration leading to reward discrepancies
Use off-chain backups, real-time monitoring, blockchain support team, and dispute resolution processes.
4.
Overreliance on the app leading to decreased authentic exploration and increased screen time
Set usage limits, include mindfulness features, encourage app-free challenges, and provide user activity reports.
5.
Potential for location spoofing to artificially claim rewards or complete challenges
Use multi-point location verification, ML to detect anomalies, photo verification for high-value rewards, and a reputation system.
6.
Economic imbalance leading to oversaturation of rewards in certain areas
Use dynamic reward allocation, geofencing, balance rewards by economic factors, and incentivize underserved areas.
TruWorld Pricing Strategy and Business Model
User Freemium Model:
-
Basic features available for free
-
Premium features accessible through paid subscription
-
Drives user acquisition and engagement
Pay-Per-Visit Promotions:
-
Businesses charged only when users physically visit
-
Aligns costs with actual foot traffic
-
Incentivizes creation of compelling offers
Business Tiered Subscriptions:
-
Bronze, Silver, and Gold levels
-
Varying degrees of exposure and features
-
Encourages businesses to invest in higher tiers
TRU Token Economy:
-
Revenue generated through transaction fees
-
Encourages active participation in the ecosystem
Strategic Partnerships:
-
Revenue-sharing agreements with tourism boards and event organizers
-
Expands platform reach and value proposition
TruWorld Lean Canvas Overview
Problem Siatement
-
Digital overload and lack of real-world engagement
-
Difficulty in discovering local businesses and experiences
-
Ineffective, costly marketing for small businesses
Solution
-
Gamified real-world exploration with 'fog of war' map
-
Blockchain-powered rewards for physical activities
-
Location-based 'airdrops' for businesses
Existing Alternatives
-
Traditional fitness apps (lack gamification & Motivation system)
-
Social media check-ins (No rewards)
-
Local business directories (static, non-interactive)
Key Metrics
-
Daily Active Users (DAU)
-
Area uncovered per user
-
Business sign-ups and retention
-
User-to-business interaction rate
Unique Value Proposition
TruWorld gamifies real-world exploration, bridging digital engagement with physical experiences while connecting users to local communities and businesses.
Unfair Advantage
Proprietary algorithm combining blockchain, gamification, and location-based services creates a unique, hard-to-replicate ecosystem.
Customer Segments
-
Individuals seeking to blend digital and real-world experiences
-
Local businesses aiming to enhance visibility and attract foot traffic
Early Adpoters
-
16-35 Tech-savvy millennials seeking a balanced lifestyle
-
Local small Brick-Mortar business owners struggling with digital marketing
-
Community-focused individuals and groups
Cost Structure
-
App development and maintenance
-
Blockchain infrastructure
-
Marketing and user acquisition
-
Customer support5. Business development team
Revenue Streams
-
Premium user subscriptions
-
Business tiered subscriptions
-
Transaction fees from token economy
-
Pay-per-visit business promotions
-
Strategic partnerships and collaborations
High-level Concept
TruWorld = Pokémon GO for real-world exploration and local business discovery
Channels
-
App stores (iOS and Android)
-
Social media marketing
-
Local business partnerships
-
Influencer collaborations
AI Scalability
TruWorld is designed with AI scalability in mind, ensuring that our location-based recommendation systems and reward mechanisms continue to perform effectively as we expand across different cities and user segments.
We have implemented regular fine-tuning of our models to adapt to location pattern shifts, allowing the AI to maintain 90% conversion accuracy even as footfall patterns and business demographics evolve. Our automated retraining pipeline uses MongoDB's geospatial indexing and Elasticsearch's geographical features to handle increasing data volumes efficiently.
To mitigate AI bias and reduce incorrect location recommendations, we employ:
-
Diverse training data from multiple urban environments
-
Continuous monitoring of recommendation accuracy across different demographics
-
Regular validation of store visit patterns against baseline metrics
-
A/B testing of reward placement strategies across various locations
This approach enables our AI to deliver personalized, reliable, and scalable location recommendations while adapting to:
-
Seasonal changes in foot traffic
-
Different business types and operating hours
-
Varying urban density and movement patterns
-
Cultural and regional preferences in different markets
